How Deep Learning Could Improve Li-ion Electrodes
| 23-11-2021 | By Liam Critchley
Lithium-ion (Li-ion) batteries are the most dominant rechargeable battery worldwide, but they don’t have the highest energy density of all commercially available batteries. The key to their success has been their relatively high energy density coupled with their long-term stability, so there is still room for improvement in modern-day Li-ion batteries. This is why we are still seeing new Li-ion batteries coming out that are better than their predecessors.
The performance of Li-ion batteries is closely linked with the electrochemical properties of the materials within them and their morphology. For example, the pore structure of the electrodes and the separator within the battery are responsible for the effective transport coefficient of lithium ions within the electrolyte. If the effective transport is low in the battery, this can impact the ionic resistance and lead to overpotentials occurring. Another example is the distribution of the binder materials around the active particles, as this dynamic is crucial for ensuring that the battery has a low electronic resistance and high mechanical stability throughout its cycle life.
The ability to tune the structure of the electrode is important for improving both their performance and application scope. Accurate 3D representations of Li-ion battery electrodes, where the active particles, binder and pore phases are distinguished and labelled, can be used to tune and improve the performance of a battery. However, the use of imaging techniques alone has some limitations, and deep learning methods are now being used to assist different imaging methods and provide a better understanding of the ways that Li-ion performance can be improved.
Taking Images of Li-ion Electrodes
The ability to produce an accurate 3D representation of the electrodes helps to choose the suitable materials, manufacturing processes and operation parameters for each Li-ion battery. Several different imaging methods are typically used to produce these 3D images, including electron, neutron and x-ray imaging techniques. Out of all these, the most common method used today is X-ray tomography, primarily because it offers a range of resolutions and can be used non-destructively to monitor the evolution of the internal electrode structure over time.
However, there are currently a few issues when trying to obtain 3D reconstructions of Li-ion electrodes. The biggest challenge is the inability to accurately segment and quantitatively analyse the images. This issue has arisen because there are differing length scales in Li-ion electrodes. There’s typically a low contrast between the key components and low attenuation of the carbon-based materials used in the electrodes.
Several different active particles have been used to provide good contrast in absorption-based imaging methods. However, graphite (the most common anode material in Li-ion materials) doesn’t allow this contrast to occur, so segmentation errors can often occur. Beyond this, many high-resolution techniques have limited fields of view, so only a few particles can be imaged quantitatively, making it harder to understand the broader picture of the electrode.
Using Deep Learning for Li-ion electrode images
It’s thought that deep learning methods can overcome some of the segmentation and resolution issues when imaging Li-ion electrodes. Deep learning algorithms have already been employed within the battery space to assess the health of a battery, improve the microstructural design and detect any defects during manufacturing.
Some algorithms are already starting to be used to segment the microstructure of an electrode. Algorithms can detect any cracks in the electrode and analyse if there is any particle detachment within the electrode’s structure. However, many of these currently rely on using heavily adapted filters, so most of the work to date has focused on high contrast systems (i.e., on cathodes, not anodes). Moreover, complete 3D segmentation approaches have not yet been applied to lithium-ion electrodes, such as those used in medical analyses.
Overcoming the Common Challenges
A new approach has now been trialled. A 3D U-net neural network architecture has been trained to segment and reconstruct (in 3D) electrodes into all their different material components—such as the binders, the pores, and the active particles. The team used volumetric images of graphite-silicon composite anodes captured by X-ray tomographic microscopy (XTM) to obtain these reconstructions. The data used in this study contained a lot of the challenges faced with image segmentation in Li-ion batteries, including multiple heterogeneous material phases with different feature sizes and low contrast between phases.
One challenge for neural networks, such as 3D U-net, is the need for the algorithm to learn from real-world data. There is a lack of real-world data because obtaining some of this data is complex and time-consuming. The team used a hybrid data set composed of both real-world and theoretically computed data points to overcome this.
Using this hybrid data set, the team was able to computationally generate a basic electrode structure utilising the knowledge of the volume percents of the different phases and the size and shape distributions of the different particles in the electrode. High quality segmented and labelled data from multi-modal imaging methods were then used to convert the basic electrode structures into a realistically looking microstructure. The electrode then underwent a tomographic reconstruction which improved the accuracy of the different electrode material features, including the pore space, graphite and silicon particles, and the carbon black-binder domain.
Because the researchers had an automated and reliable segmentation tool, it enabled them to address the trade-off between an obtainable resolution and the image volume. This allowed them to work with small sample sizes at small length scales (for quantitative imaging purposes). Still, they could image and segment multiple electrode samples to obtain a much larger volume. This larger electrode volume could then be used to provide statistical insights into the structure of the different material phases at different length scales.
Another aspect of the study looked at how these segmentations could provide insight into the microstructural evolution of the composite anodes over time as a function of their electrochemical cycling. This part of the study was able to show that in a graphite-silicon composite anode, a large number of the morphological changes to the structure of the carbon black binder occur around the silicon particles and not in the localised regions where there are graphite particles.
Overall, this approach has allowed Li-ion electrodes to be probed in more detail at the microstructural level. This has been tricky because there has typically been a lack of available data—and provide new insights into how the structure of these electrodes change over time. The ability to combine real and computed datasets to create an accurate model (using the volume fraction of each material phase in the electrode) has the potential to offer a new way for Li-ion battery manufacturers to better analyse and improve their electrodes at the fundamental level (i.e., micro and nanomaterial scale).
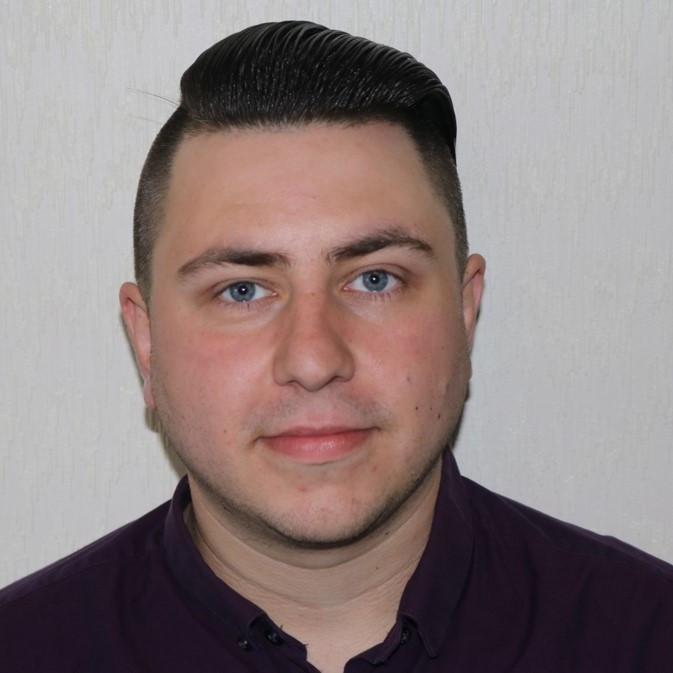