How Machine Learning Has Helped Develop Computational Chemistry
| 27-10-2020 | By Liam Critchley
In years gone by, chemical research solely focused on trial and error experimental approaches using prior knowledge gained from personal experience and the literature. While there has always been a lot of reactions and chemical pathways in the literature that scientists can use, there are a lot of unpredictable and spontaneous scenarios that can happen during a reaction/series of reactions. This is one of the reasons why chemical research and development needs time and patience to produce results.
Over the last decade or so, the field of computational chemistry has been growing. While it was around long before that, it’s used was niche and limited, but it has become a very powerful tool for optimising and predicting chemical research in recent years. Using computational methods, chemists can now predict how reactions might work, the best parameters, reagents and reaction conditions to use, and they can even use computational methods to predict the structure and properties of the material/molecule they are planning to make.
So, computational methods can aid the chemist throughout the whole conception, development and analysis processes. So, why has the use grown in recent years? For one, chemists have become more understanding of computational chemistry and realised the benefits it can have. Two, the computing power needed to power these simulations is now more readily accessible to more scientists. Thirdly, and finally, advances in machine learning algorithms and their integration into computational chemistry processes has enabled more accurate results to be obtained—which have a higher probability of success when performed experimentally.
Applying Machine Learning to Chemical Processes
Like many areas where machine learning is being implemented, its use in the field of computational chemistry is to take all the known data from the literature, extrapolate and analyse it, and predict the most likely outcomes. For the chemical sector, this often means taking the data from different reactions, such as the types reagents, the concentrations of chemicals, the process conditions, as well as the products that can be made.
All this data is valuable, as they are all factors that can determine the outcome, making the reactants an ideal set of inputs and the products the output. The use of this data can be fed into the machine learning algorithm and can be used to do three key things. The first is that by using prior data, it can extrapolate the most likely reasons for a chemical structure forming (from a reaction/process perspective) and this can be used by industry to predict new molecules that perform their desired function.
The second way is more to do with the processes themselves. Sometimes, researchers have a product in mind but don’t know the process. The data can be taken from previous reactions and analyzed, which enables the algorithms to predict which conditions and reagents would be responsible for the formation of different chemical groups in a molecule. This enables reaction pathways to be created by the algorithm, which shows the most likely route for building the molecule step by step.
The third way is a complete molecular design approach which starts with an idea but no defined product or reaction pathways. This takes the principles of the other two points. Still, instead of one variable (product or reaction), both are technically unknown, so the algorithms need to extrapolate both the product and the reaction conditions to produce likely outcomes/pathways. This is a harder task to perform but one that is receiving a lot of attention.
Machine Learning to Predict Molecules
The other main arm of computational chemistry is in the prediction of the materials/molecules themselves, their basic intrinsic properties, and how they might behave in certain scenarios/environments. This has been the more fundamental and longer-time use of computational chemistry and is often used more in academia when investigating new materials and molecules, compared to process optimisation which is typically found in industry (as this is where time, money and efficient product scale-up and output are key). It should be noted that these efforts are not limited to just the chemical sectors, as similar computational methods are using in the biological and engineering spaces as well.
Even though there are fewer factors to focus on (i.e. just looking at the molecules rather than both the processes and the molecules), the use of computational chemistry in this area is important as it helps to achieve results at the fundamental level—and this is often the stage that happens before the industrial processes are created—and machine learning has also really helped to elevate this area as well.
Simulating the structure of a molecule and how it performs is no easy task. It has been limited over the years by the sheer number of variables that need to calculated vs the computational power available (many researchers share a supercomputer to perform said calculations). Machine learning has really helped in this regard as the various amounts of atomic arrangements, bond energies, energy and reaction barriers, quantum properties, magnetic and excited molecular states, and inter and intramolecular interactions can all be computed with greater ease than before.
Extrapolating and predicting the best solutions from a set of variables and known data points is what machine learning does best, meaning that the vast amount of data that has to be computed can be optimised much more easily using machine learning algorithms. Many of the above variables have a significant bearing on the structure and properties of a molecule/molecular system, and this has led to much more accurate molecules and properties being deduced than in previous years. It has even enabled trickier atoms—such as the d and f block elements of the periodic table—to be computed with a high degree of accuracy, whereas this was not possible in years gone by.
Conclusion
Even though there are several different computational programs used to create these molecular simulations, machine learning can be applied across them all. Machine learning has helped to not only optimise and improve chemical and drug discovery processes at the industrial level, but it has also played a key role at the fundamental level for deducing molecular structures and properties of known and unknown molecules, understanding how molecules behave in certain situations, and what the most likely outcomes of a reaction will be.
Overall, machine learning has had a tremendous impact on computational chemistry already and is likely to do more so in the coming years as more chemists are turning to computations/simulations first before trying experimental procedures.
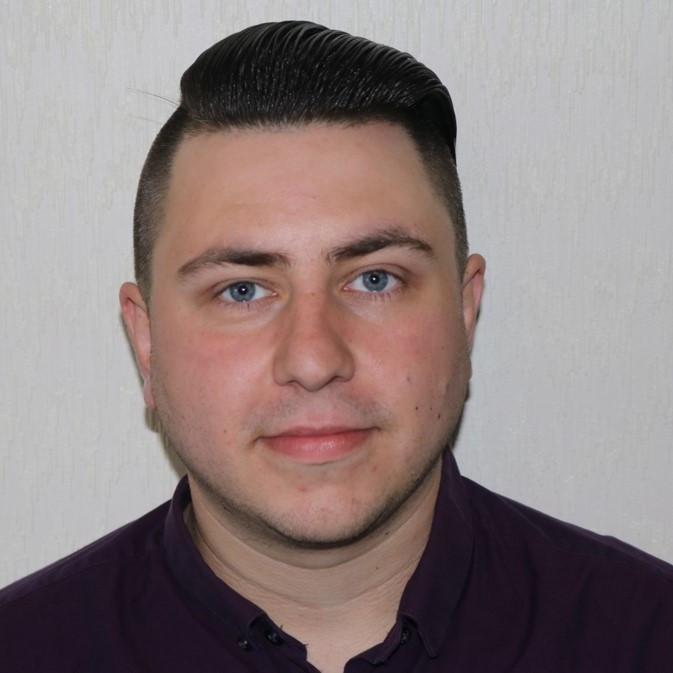