Probing the Bioactivity Characteristics of Chemicals with Neural Networks
| 20-07-2021 | By Liam Critchley
Over the years, computers and data science methodologies have increased significantly within the chemical sciences (and the more expansive application areas associated with chemicals). A lot of this has centred around theoretical chemistry, molecular simulations and computations designed to elucidate structures and properties of different chemical species. In recent years, there has also been a growing interest in cheminformatics.
As it stands, despite all the information that we have about different chemicals and molecular species, there are still large parts of the chemical space that remain unidentified. This is particularly true when trying to understand how other chemical species can be used in biological settings. As drug development and medicinal chemistry have become some of the more general areas of organic chemistry over the years, there is a growing interest in identifying how different chemical species will behave in biological environments.
Cheminformatics and Cataloguing the Chemical Space
Cheminformatics is a way of using computational resources to solve practical chemical problems and transforms data into extractable information. Compared to other computational chemistry methods, it uses actual physical data to provide insights rather than predicting chemical structures and properties based on best theories.
Several different cheminformatic branches are used today, including storing and retrieving chemical information, acting as a chemical information library, and as a screening tool (using the stored data) to determine which chemical species may possess biological activity. It’s a valuable field to the chemical sciences, especially the industrial side, as it allows both time and costs to be saved compared to utilising multiple trial and error experimental methods.
For cheminformatics to be valid, it needs access to large amounts of data. Because the chemical space is so vast, scientists have invented a range of chemical descriptors which encode these physicochemical and structural properties of small molecules—and molecular fingerprints are a widespread form of chemical descriptors to determine different substructures of chemicals.
The descriptors are a fundamental part of cheminformatics and allow compound similarity, clustering, computational drug discovery (CDD), structure optimisation, and target prediction operations to be performed using the data. For drug discovery applications, bioactivity properties are also key, and with the data available, it has emerged that the bioactivity of molecules can be deduced using other numerical representations that capture the known biological properties of different molecules. This is through using bioactivity signatures.
From a cheminformatics perspective, bioactivity signatures are multi-dimensional vectors that capture the different biological characteristics and traits of a molecule. These signatures are processed into a format compatible with the structural descriptors and molecular fingerprints typically used in cheminformatics. The first biological descriptors captured ligand-binding affinities and the target profiles of small molecules, which revealed several previously unknown associations, and this has since been used as a starting place to build upon the bioactivity properties of different molecules.
Building a Useful Chemical Database
As it stands, the publicly available chemical databases only have experimentally determined bioactivity data for around a million molecules, and while this may sound a lot, it is only a small percentage of commercially available chemical compounds. This means that bioactivity signatures/bioactivity descriptors are not available for most compounds. In practical terms, this limits computational drug discovery methods as the information available to these operations regarding the bioactivity of different molecules is limited.
The team recently integrated the major chemogenomic and drug databases known to researchers into a single system named the Chemical Checker. In doing so, they created the most extensive collection of small-molecule bioactivity signatures to date. In the Chemical Checker, the different bioactivity signatures are organised by data type—e.g., toxicology profile, cell sensitivity—and follows a chemistry-to-clinics rationale that enables relevant signature classes to be selected at each step of the drug discovery pipeline.
The chemical checker is a different way of representing all the small-molecule knowledge in the public domain. While it is helpful to aggregate all this information into a central system, the database is limited by the availability of experimental data, much like the other databases. The database is more useful when substantial amounts of bioactivity data are available for each molecule, so it is still limited for poorly characterised compounds.
Understanding Bioactivity of Compounds with Neural Networks
In an industry where bioactivity is a crucial parameter, a lack of knowledge hinders the performance of these computational operations, but AI neural networks could offer a way to overcome this. Using their previously constructed Chemical Checker database, researchers have now used a cluster of deep neural networks, specifically Siamese neural networks, to deduce bioactivity signatures for any compound of interest, even when little or no experimental information is available for them.
The research team observed that the different bioactivity spaces within the signatures are not entirely independent, so it was deduced that there are similarities within each given bioactivity type. It transpired that these similarities within signature types can be transferred to other data sets.
The approach enabled signatures to be interpreted at a coarser level, indicating which signatures were more informative for different predictive tasks. The current study only looked at 25 different signature types, but this still provided enough predictive information of other bioactivity properties for them to be used as drop-in replacements for chemical descriptors in day-to-day cheminformatics tasks. Because of how the neural networks work, the chemical descriptors in the Chemical Checker database are likely to evolve and change, but the researchers have stated that they will release updated signaturizers for the database each year.
Beyond day-to-day tasks, the researchers looked at the ability of the neural network to use these predictive signatures on a mostly uncharacterised compound library. This was done by identifying compounds against the drug-orphan target, Snail1, and implementing a battery of signature–activity relationship (SigAR) models for predicting the biophysical properties of the molecules.
This approach enabled bioactivity signatures to be generated for the compounds in the database that had unknown signatures. This kind of AI analysis (using the signature similarities) can be taken forward for predicting the bioactivity of uncharacterised molecules.
Overall Outlook
While no computational tool is perfect without physical, experimental data, the approach here enables a first estimation of the biological properties of different compounds to take place—using the similarities within different signature types—allowing the researchers to have an idea as to whether there is the potential for a particular compound to be helpful for a specific drug approach (and investigate further if so).
Because bioactivity signatures can now be made available for any compound, within a relative degree of confidence, the Chemical Checker database could become a reference tool for drug discovery applications to scrutinise the expected bioactivity of a compound and to see if it warrants further interest and research, or whether other options may be better.
The more computational tools available to pharmaceutical companies and drug discovery researchers, the better the decision can be made without excessive trial-and-error approaches being undertaken, which reduces the time and cost to bring new drugs and therapies to market. More tools are always better, and in many cases, they are used in conjunction with other computational methods, so wherever there’s a shortfall in information in one method, another process can often be used to fill in the gaps—so the more tools, the better.
Reference:
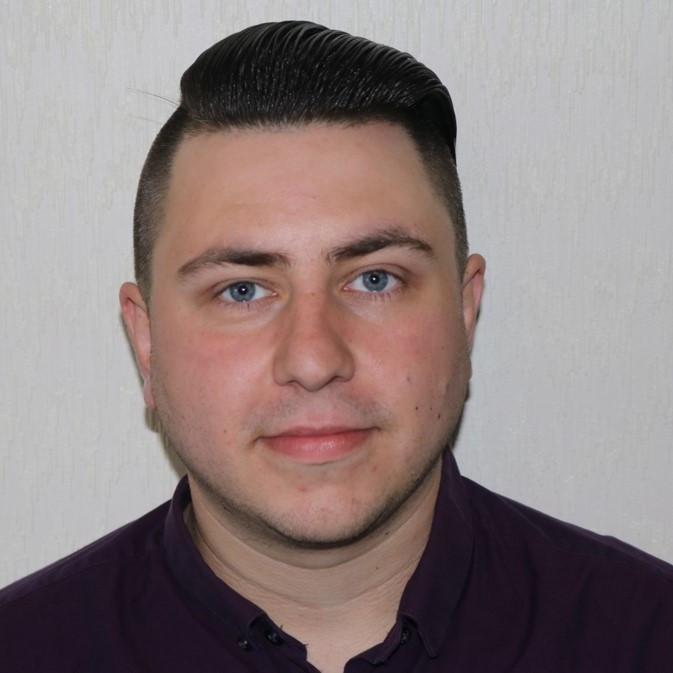