Machine Learning Improves Hydraulic Turbine Lifespan
Technical Analysis | 15-05-2025 | By Liam Critchley
Key Takeaways:
- Hydropower's role is growing – As intermittent renewables increase, hydropower offers essential grid support through stable frequency and voltage regulation.
- Fatigue damage is a rising concern – Modern turbines face far more startup cycles than older systems, accelerating fatigue and wear on components like runner blades.
- Machine learning is redefining turbine operation – A new model trained on experimental data identifies optimised startup sequences, reducing fatigue by up to 99.5%.
- Scalable solutions for future plants – Though tested on reduced-scale models, the approach shows strong potential for full-scale hydropower deployment and predictive maintenance.
The world is shifting towards more renewable energy systems being integrated into the grid. Renewable energy integration is now accelerating at a fast rate, and there are further ambitious efforts set aside for the future. For example, the European Union (EU) has set a target of having at least 42.5% of its energy generation capacity coming from renewables by 2030, with aspirations to make it above 45%. These policies being implemented are part of a global trend towards decarbonising the grid to reduce greenhouse gas emissions.
The acceleration that the renewable energy sector is experiencing means that achieving high stability in power systems is going to be even more crucial than before. As more renewable energy systems are integrated into the grid, the transition to a decarbonised energy network will require robust support systems to maintain frequency and voltage stability, since most new sources, such as solar and wind, are intermittent and prone to fluctuations in power generation.
One renewable energy source that is not intermittent is hydropower. Hydropower now accounts for around 17% of energy production worldwide and is well-suited for providing ancillary services and added resilience to the grid because it can provide both active and reactive power regulation. The hydraulic turbines have always been optimised for high performance during steady-state operations under constant control parameters. With there being a potential shift in how hydropower systems might be utilised in the future, developing new turbines that are safer and last longer is going to be crucial for providing grid support services. It’s thought that machine learning can help with the design of new hydraulic turbines.
The Demand for Hydropower Set to Rise―Fatigue Damage Becomes A Worry
The fluctuating loads and mechanical stresses that hydraulic machines undergo during multiple startup operations can cause fatigue damage. With the demand for ancillary services projected to increase in the coming decades, it will change the operating schedules of hydraulic turbines. Hydropower systems need to adapt so that they can better handle these off-design and dynamically changing operation conditions, as more frequent startup sequences cause dynamic loads that lead to fatigue damage.
Over the years, hydraulic turbine design has not really considered fatigue damage caused by startup sequences, as they typically undergo few startup sequences during current operations. So, the effects were considered insignificant. Turbines have been designed to handle a few dozen start-stop cycles per year. However, with the need to provide more ancillary grid services, the number of cycles could be up to 500 a year, which means that fatigue damage needs to be considered to ensure that hydropower stations remain safe during operation.
Fatigue Damage in Hydraulic Turbines
Fatigue damage is the degradation of a material’s structural properties to the point where cracks form and propagate throughout the material, leading to structural instability that can cause the turbine to fail. Hydraulic turbines are typically formed under cyclic and fluctuating stresses but first originate during manufacturing (during casting or welding). Once the cracks have initially formed during manufacturing, if the hydraulic turbines are exposed to prolonged high-loading conditions, then cracks can propagate and develop into more significant macroscale cracks that affect turbine performance.
While many components in hydraulic turbines are exposed to dynamically fluctuating stresses during operation, stresses caused by fluid-structure interactions and vibrations cause the most damage. The components that are often worst affected by these interactions are the runner blades because they undergo the most intense loading.
The impacts of fluid-structure interactions and vibrations are dependent on the operating sequences of the hydraulic turbines, including the startup trajectory, turbine rotational speed and the guide vanes opening angle (GVO). Modernising hydropower systems will hinge on being able to better predict these stresses and the induced fatigue, so that operations can be optimised, and maintenance can be better planned.
Limitations of Traditional Fatigue Analysis in Hydropower
Reduced-scale physical models are currently used to verify that a turbine design prototype will meet its expected performance, estimate local damage, and predict turbine lifespan. During these tests, the turbine speed, GVO and other control parameters are continuously measured alongside the induced stress in the turbine. The damage from the fatigue can then be estimated, but the process is both expensive and often inaccurate due to the complex geometry of turbines, which makes it difficult to create accurate models.
Data driven methods are now enabling engineers to develop more accurate models for analysing the stress in turbines, based on the control parameters used and experimental data generated during these tests. Using data driven methods is helping to provide more accurate insights into the lifespan of hydraulic turbines.
Data-driven methods have already shown that transient start-stop sequences at a low discharge operation can cause severe degradation that’s equivalent to multiple days’ worth of extra fatigue compared to normal operating conditions. Studies have shown that the fatigue damage is due to stress alteration on the turbine blades during startup, while other studies have shown that modifying the startup schedule can reduce fatigue damage and extend the life of the turbines.
Machine Learning Models for Predicting Turbine Fatigue
With these consideration in mind, researchers have now developed a new data-driven machine learning-based approach that identifies identify the best transient startup trajectories to minimise fatigue damage. The machine learning models were trained on experimental stress data from reduced-scale model turbines.
This approach responds to operational demands in modern power systems, where frequent turbine activations have increased from a few dozen annually to hundreds of cycles due to the variability of renewable energy inputs. The machine learning model was built using experimental data from start-up trajectories—including Classic, Linear, 2Slopes, and BEP patterns—to identify fatigue-sensitive operational sequences.
The researchers used an input time-frequency deep neural network (iTF-DNN) model. The training data came from several startup trajectory measurements from an experimental campaign that complied with IEC standards. The measurements obtained in the experimental campaign were mechanically and hydraulically homologous to the full-scale hydraulic machine at the Hydraulic Machines Platform at the Swiss Federal Institute of Technology Lausanne (EPFL-PTMH).
The iTF-DNN model captured both slowly varying stress trends and high-frequency oscillations, trained on real measurements from turbine components such as runner blades. By modelling stress in both the time and frequency domains, the neural network achieved prediction accuracy exceeding R² of 0.97 across training and validation datasets.
The fatigue damage was estimated based on the predictions made using the iTF-DNN model alongside Rainflow-counting and Miner’s rule approaches. The researchers then used pathfinding techniques to optimise startup control trajectories and predict the level of failure in the turbine. Once optimisation schedules had been deduced, the researchers put them into practice on a reduced-scale model at EPFL-PTHM in a secondary experimental campaign and analysed the induced stresses under these optimised startup trajectories and compared them to the original non-optimised experimental results.
The optimisation was carried out using Dijkstra’s algorithm, which translated the fatigue minimisation challenge into a shortest-path problem. This enabled efficient trajectory discovery within predefined turbine safety parameters, such as guide vane angular velocity and turbine acceleration constraints.
Results and Real-World Applicability of Fatigue Optimisation
The observations and results from this secondary experimental program showed that the optimised startup trajectories can significantly reduce the accumulated fatigue damage during machine startup. The optimised trajectory deduced by the machine learning model achieved an average damage reduction of over 99.5% compared to conventionally used trajectories. Other optimisation trajectories have been proffered in the past as an alternative, but this new machine learning-enhanced trajectory also had over 70% average damage reduction compared to those trajectories as well.
In real-world terms, this means that using a conventional start-up sequence can cause fatigue equivalent to more than 100 days of operation at the best efficiency point (BEP). In contrast, the optimised trajectory incurred only the equivalent of 9.5 hours, representing a substantial reduction in accumulated mechanical stress.
Reduced-scale models can’t fully replicate the full-scale system, including the full complete hydraulic response of a hydropower station―especially the pressure that builds up and the vibrations that affect the shaft and casing―due to how complex and interconnected many of these components are. However, the runner blades are one of the biggest areas of potential fatigue, and the results from machine-learning enhanced reduced-scale models can be adapted to full-scale models with good accuracy.
Although reduced-scale platforms do not fully replicate hydraulic responses such as shaft vibration and pressure pulsations, the stress patterns on runner blades align closely with full-scale machines. This lends confidence to the scalability of machine learning-based fatigue optimisation frameworks in operational hydropower plants.
The model has shown a way to effectively reduce the wear and degradation of hydropower turbine blades of the new start-stop operations they will need to undergo more frequently in the coming years. The optimised model minimised the damage during startup, which will increase the lifespan of hydraulic turbines in coming years as they are put under new stresses and strains. The study paves the way for validating full-scale hydropower plants so that they can be ready for the energy challenges ahead and offer a way to improve hydraulic turbine design to meet future energy goals, as well as improve hydropower operations and maintenance schedules.
By integrating stress prediction into the design and control logic of hydraulic systems, turbine life cycle management can shift from reactive maintenance to predictive optimisation. The study sets a precedent for coupling AI techniques with experimental turbine data to create self-adaptive operational strategies for renewable energy infrastructure.
Reference:
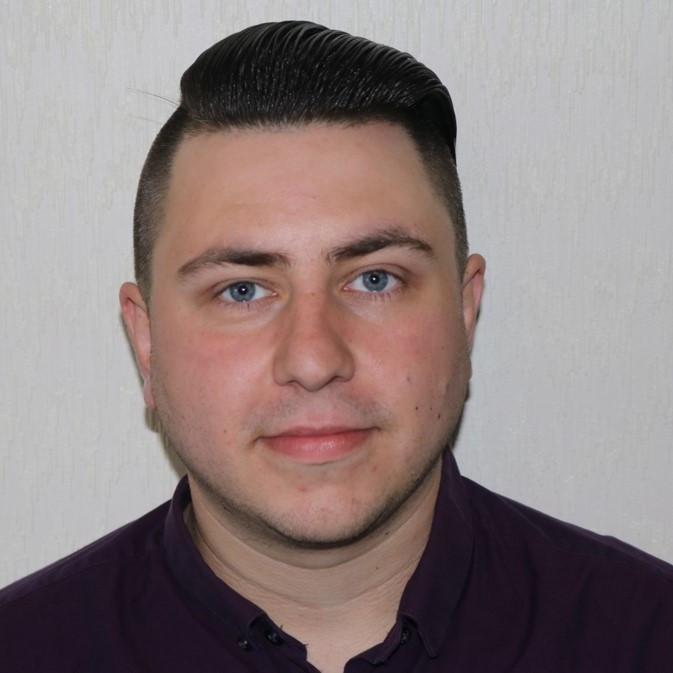