Combining Machine Learning with DNA-Storage Approaches
| 21-06-2022 | By Liam Critchley
DNA-based storage technologies have gathered much interest in recent years as a non-volatile memory device. Nature is known to be the world’s best scientist and can create structures and operations that are a major struggle to replicate synthetically, so there is a lot to be learned from these systems. DNA in its natural environment can encode and store a vast amount of information, and as the need for data storage gets greater in the modern-day data-driven society, scientists and engineers are looking at bioelectronic options beyond the all-synthetic storage devices that we use today.
It’s safe to say that DNA-based storage is nowhere near the level of the synthetic storage technologies used today, but a lot of research is being undertaken that is slowly advancing the field and improving the performance of the devices. One of the latest developments has been to move away from the traditional architecture seen with many DNA-storage devices to create a system that can utilise machine learning algorithms to encode, decode, process, and store images and image-based data.
Traditional DNA-Storage Systems
DNA-based storage devices are increasingly being seen as a viable alternative to the classical magnetic, optical, and flash memory devices used in today’s electronics. Many DNA-based storage device architectures to date store the user information in synthesised DNA strings (oligos) and retrieve the data via either high-throughput sequencing technologies or nanopore sequencing.
Even though there has been a lot of progress in the design of DNA storage devices, they typically only encode information in the nucleotide sequence of the molecule, leading to some issues that could make them more impractical on a commercial level. Some of the key issues to date include the high cost of synthetic DNA, the lack of a simple rewriting mechanism, large read-write latencies, and some missing oligo errors.
When it comes to images, the image data often needs to be compressed before being recorded, so a single mismatch can lead to a very large error during decompression, ultimately leading to an unrecognisable reproduction of the original data. Other issues include sequencing errors varying in magnitude from one platform to another, as well as PCR reactions and data rewriting operations causing a gradual increase in sequencing errors.
In many data storage systems, ensuring an accurate reconstruction is enabled by accounting for the worst-case scenario and performing extensive read-write experiments to determine the error rates of the device―before adding in a redundancy for these errors. However, the nature of many DNA-based storage devices makes it difficult to obtain an estimation of the error, leading to large errors occurring in some devices. To overcome the challenges of many DNA-based memory devices, a change in architecture has been proposed toward a hybrid model where the information is recorded in both the sequence and backbone structures of the DNA.
Creating Hybrid Devices that Utilise Machine Learning
Researchers have now created a 2D DNA-based hybrid storage device that records information in both sequence and backbone structures of the DNA, allowing it to perform joint data encoding, decoding, and processing operations. The name of the device has been termed the ‘2DDNA’ and has been developed to primarily tackle the issues surrounding rewriting and to avoid the use of worst-case error-correcting approaches that are typically needed to compensate for missing and random oligos in the infrastructure of the device.
The 2DDNA utilises two different information systems to combine the desirable features of both synthetic and nick-based recorders. On the one hand, the images are stored in the synthetic DNA, but the metadata for the sequence-encoded images (ownership information, dates, clinical status descriptions) is superimposed and stored as nicks in the DNA backbone.
The sequences contain a lot of information, but such a large amount of information has been the cause of poor rewriting operations in many DNA-based devices. However, information stored in nicks is typically smaller in volume and is much more suitable for efficient, permanent and privacy-preserving erasing and rewriting operations. Moreover, the information in both the sequence and the backbone can be read simultaneously.
The other challenge was to avoid using worst-case scenario redundancy for correcting errors in the sequence and/or rewriting operations. To mitigate the potential mismatch errors (in the decoding parameters), the researchers employed machine learning algorithms to detect if the stored images suffered from any discolouration or inpainting effects―which would suggest that there are issues with the data.
The machine learning approach uses a compression scheme for images that operates on three separate colour channels. Machine learning and computer vision approaches reconstruct the images and enhance the quality to generate high-quality replicas of the original image. The device was experimentally tested by reconstructing a library of images, where the images had either undetectable or very small visual degradations. The corrections were performed by erasing and rewriting copyright metadata encoded in the nicks.
The results from the study have shown that DNA can be used as both a write-once and a rewritable memory and that the data can be erased in a permanent and privacy-preserving manner. The approach tackles some key challenges of DNA-based storage devices and removes the need for worst-case redundancy approaches.
While it may still be a while off before DNA-based storage devices fall in line with other synthetic devices, this hybrid and AI-driven approach offers the possibility of retrieving images of quality and a high information density. The device provides a way to effectively rewrite the data using the metadata held in the DNA backbone and should be suitable for use (in the future) in applications that use either synthetic or natural DNA strands as the sequencing oligo for the data.
Reference:< /br>
Milenkovic O. et al., Rewritable two-dimensional DNA-based data storage with machine learning reconstruction, Nature Communications, 13, (2021), 2984
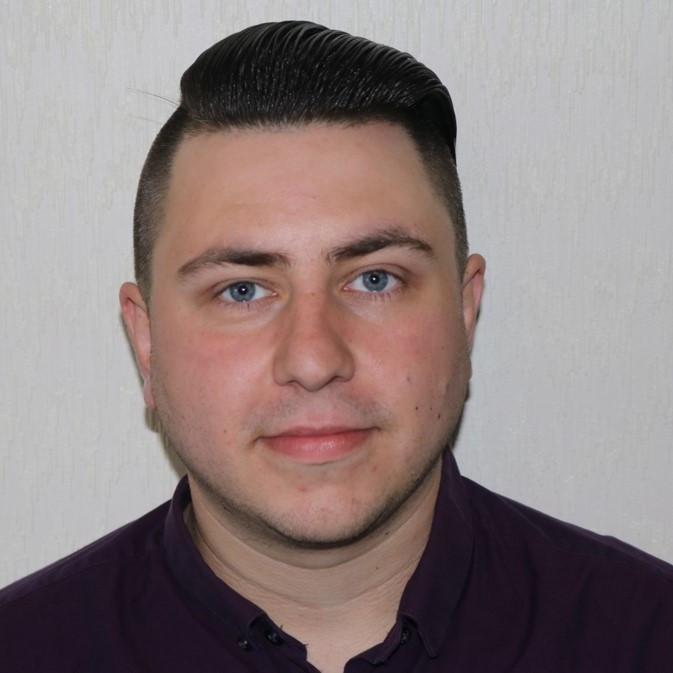