Using Graphene Memristors to Build Neuromorphic Computing Hardware
16-12-2020 | By Liam Critchley
Graphene is a material which has been touted for use in a wide range of applications and industries, including many within the electronics sector. A lot of progress is being made at a fundamental level in many areas. Still, the commercialisation of graphene in some electronic applications is going to take a while—mainly because the long-term stability and performance still need to evaluate against the status quo (where in many cases, the same materials have been used for many years).
Nevertheless, innovation is happening on many levels, and interesting applications involving graphene are constantly appearing. One of the latest areas, and something a bit different to other areas (even within electronics), has been to use graphene to create memristors for neuromorphic computing hardware—which are electronic circuits and electronic architectures that mimic neuro-biological architectures present within the human nervous system.
Memristors are now becoming a vital piece for computer architecture across in-memory computing engines for artificial neural networks (ANNs)—and ANNs are themselves becoming a set of powerful artificial intelligence (AI) algorithms for many areas of automation. While complementary metal-oxide-semiconductor (CMOS) architectures have been the gold-standard for many years, engineers are looking at other alternatives, and the human brain (and its attached central nervous system) has been gathering interest for AI-related applications.
How ANN’s Can Emulate the Human Brain
It goes without saying that having a higher computational power helps AI algorithms to perform their operations more efficiently. Even with modern computing advances, the supercomputers available nowadays are nowhere near close to rivalling the brain when it comes to energy and area efficiency as the brain can perform significantly more operations per watt. So, if the power of our very own ‘natural computers’ could be harnessed, then our man-made computing and AI operations could potentially become much more efficient.
Work has been done in emulating the brain and ANNs have become one of the best methods to do so, because the ANNs can be used to mimic one of the most fundamental aspects of the human brain—the electrical synapse. One of the key aspects to mimicking the brain is the ability to adapt to external stimuli, which varies over time, and ANNs have been able to do this to some extent by modulating the synaptic weights that are connected to the artificial synapses, as this allows the connectivity of the whole ANN system to be reconfigured with changing environments.
These artificial neural reconfigurations can reproduce the biological functionality of real neurons by using devices that can change their resistance/conductance (which acts as the synaptic weight) when there is a synaptic activity—which takes the form of an applied current or electrical bias. These devices also require several different resistance/conductance states to function effectively. Memristors have become one of the devices that are being used to facilitate the synapses within these neuromorphic computing architectures.
Current Challenges with Building Neuromorphic Systems
Even though ANNs have shown a lot of promise, they can’t yet be scaled up to the full capacity of a human brain without becoming power hungry and area-inefficient, because some of the current architectures separate logic and memory physically, making its scalability limited. When it comes to compatibility with existing CMOS technology, the process becomes highly inefficient, and it is worse with some devices compared to others.
This has been remedied somewhat by using crossbar architectures, such as memristors, where each of the conductance states acts as a non-volatile memory cell. As this reduces the data shuttling bottleneck between the memory and the compute seen in other ANN neuromorphic architectures. While this helps to increase efficiencies, most memristors are binary in nature as they typically possess two resistance states—a high resistance state where the device is ‘off’ and a low resistance state where it is ‘on’.
An analog operation (with multiple resistance states) is preferable to binary operations as more operations minimise the quantisation error, leading to more accurate and efficient systems. However, achieving multiple resistance states is difficult within many memristors, and approaches to implement analog operations in binary devices has led to ANNs not being able to converge correctly. So, new approaches are being trialled to find how memristors can achieve analog operations, and recent research has turned to graphene to help.
Creation of Graphene Memristors for Neuromorphic Computing
Recent research into graphene memristors has led to some interesting results for these synaptic ANN systems and neuromorphic computer hardware in general. Based on programmable graphene field-effect transistors (GFETs), the result was a resistive memory device capable of achieving more than 16 conductance states, solving some of the key issues with existing architectures.
This is a significant improvement on the binary states seen with many memristor devices and is a significant achievement in the graphene memory space in itself. While graphene-based memory devices are not a new concept, most graphene memory devices only have 2 memory states (1-bit) on a single device, so the existence of multiple states is rather new and unique for this kind of architecture. The graphene memristors also showed desirable retention and switching endurance, as well as an enhanced accuracy compared to other synaptic devices.
While this is still only early days, the use of graphene presents some potential for furthering neuromorphic computing by making the ANNs more efficient and accurate. More people are trying to get graphene into the electronics and computing space, so it’s interesting to see more innovative areas come about. There is also a lot of collaboration within the graphene industry, so there should be no barriers from this side of the industry. Still, we will have to see if the data and computing sectors look further into adopting graphene memristor architecture (and graphene-enhanced memory devices in general) for advanced computing systems.
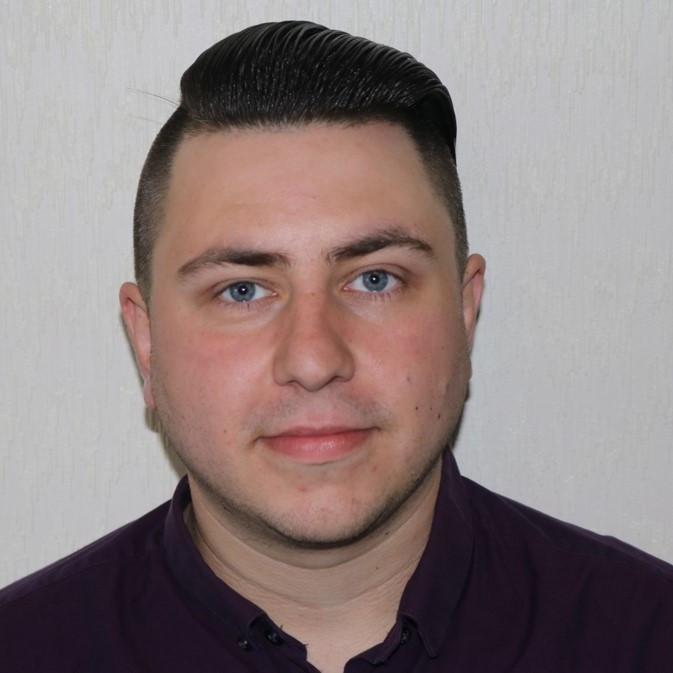