Nuclear Reactor for AI Datacenter Debuts in the US
19-05-2025 | By Robin Mitchell
Image credit: aalo.com
Artificial intelligence has become a defining force in modern technology, transforming industries and reshaping the way we live, work, and connect. Yet beneath the surface of this rapid advancement lies a pressing and often underestimated issue: energy. Training large-scale models demands enormous power, and even day-to-day inference puts a significant strain on data infrastructure.
Key Things to Know:
- AI's rapid rise is creating unprecedented energy demands – Training and inference at scale are pushing data centres to their power limits, outpacing the capacity of conventional grids and renewables.
- Aalo Atomics has launched a nuclear reactor designed for AI workloads – The Aalo Pod introduces a modular, grid-independent power source tailored for hyperscale data infrastructure and AI inference demands.
- Modularity and siting flexibility set the Aalo Pod apart – Built to scale with demand, the reactor can be deployed quickly, operates without water cooling, and supports both on- and off-grid installations.
- Nuclear energy offers a viable path to stable, sustainable AI power – Despite ongoing challenges, its reliability, zero-carbon output, and high power density make it uniquely suitable for powering AI’s next chapter.
What will it take to keep this momentum going without overwhelming our power grids or derailing sustainability efforts? And could nuclear energy provide the scalable, reliable solution AI so urgently needs?
The AI Energy Challenge
Artificial Intelligence has landed like a meteor on the modern technological landscape. Machines that can reason, interpret, generate, and even "think" have shifted everything from how we search the web to how we write code, diagnose illness, or optimize logistics. AI isn't just a trend—it's a tectonic shift. But while the world races to out-AI each other, there's a problem looming behind the scenes, one that doesn't get nearly enough press: energy. To understand how we got here, it’s worth revisiting the major turning points in AI's evolution — explored in depth in The History of AI: Key Milestones & Impact on Technology.
Training modern AI models requires an absurd amount of power. We're not talking about spinning up a couple of EC2 instances—we're talking megawatt-scale training clusters chewing through datasets the size of the written internet. And inference? It's not exactly light-duty, either, especially at scale.
Sure, we've developed efficient hardware, such as GPUs, TPUs, ASICs, and FPGAs. But while these accelerators certainly help, they still struggle to offset the sheer exponential demand curve we're riding.
Why Renewable Energy Alone Can’t Sustain AI
Now, fossil fuels have historically been the backbone of constant, on-demand energy. Like it or not, coal, gas, and nuclear kept the lights on and the servers humming. But now we're phasing many of these out (such as coal, which has no place in a clean future), and their replacements—namely solar and wind—can't handle existing workloads.
Why is this the case? Unlike most energy consumers, datacenters never stop working. Your house might draw less power at night, and your office might shut down at 6 PM, but a server farm pushing AI inference worldwide doesn't get a break when the sun sets or the wind stalls. It demands constant, stable, high-density power. Solar doesn't shine at night. Wind doesn't blow on schedule.
Some believe that grid-scale batteries are the answer to stable power . However, these are not the magic fix that many would proclaim. They're expensive, resource-intensive, and nowhere near ready for the kind of load-balancing required to power hyper-scale data centers.
Exclusive Nuclear Reactor for AI Launched in US
In a move that could significantly change the energy landscape, Aalo Atomics has launched the Aalo Pod, a nuclear reactor designed specifically for powering AI data centers. The Department of Energy has confirmed that it will be backing the project, which aims to provide clean and efficient energy for the rapidly growing industry of artificial intelligence.
The strategic push behind the Aalo Pod reflects a broader shift in how critical infrastructure is being reimagined. With hyperscale and AI-centric workloads demanding power that is not only abundant but locally controllable, the project leverages a factory-built, extra modular architecture specifically tailored for scalable deployment near data hubs. This marks a move away from the legacy model of grid-dependence and towards infrastructure co-location — where the power source and data infrastructure share a physical footprint to reduce latency and maximise operational control.
To better understand why artificial intelligence infrastructure is pushing energy systems to their limits — and why new approaches are urgently required — this short video from U.S. Department of Energy explores the dramatic rise in AI energy consumption and its broader implications:
A Scalable, Reactor-Based Power Model for Data Centres
The Aalo Pod is a modular reactor that can be scaled up or down depending on the energy needs of the data center. Each unit consists of five Aalo-1 reactors, which are cooled by sodium and paired to a single turbine, generating 50 megawatts. The reactors are designed to be compact and can be built quickly, with the goal of creating a production line that can churn out reactors like cars.
By embedding N+1 redundancy within each Aalo Pod, operators can maintain uninterrupted service even during scheduled maintenance or refuelling. This level of fault tolerance — often benchmarked at up to 99.999% reliability in multi-pod configurations — aligns with the stringent uptime requirements of AI deployments. As demand for always-on processing accelerates, particularly for inference at the edge, this type of resilience architecture will likely become a baseline expectation for next-generation facilities.
Designed for Autonomy in Demanding Environments
One of the key features of the Aalo Pod is its ability to operate independently of traditional grids, making it an ideal solution for data centers that require large amounts of energy. The use of sodium as a coolant also eliminates the need for water, which can be a significant advantage in areas where water is scarce.
This water-independent approach addresses not only environmental concerns but also strategic siting flexibility. Traditional nuclear systems often require proximity to large water bodies for cooling — limiting geographic availability. In contrast, the Aalo Pod enables deployment in arid regions or locations closer to digital infrastructure, sidestepping a common limitation of clean energy solutions and helping decentralise energy resilience for critical systems.
Flexible Deployment and Expansion for Evolving AI Demands
The Aalo Pod has been designed to be flexible, allowing it to operate in both grid-connected and off-grid modes. This flexibility gives data center operators the ability to mix and match different energy sources, depending on their needs. The reactor's modular design also allows for easy expansion, making it an ideal choice for data centers that require additional power.
The Aalo Pod’s modular strategy also allows operators to adopt a “pay as you grow” approach — adding 50MW increments as demand scales without overspending upfront. This is especially relevant for AI start-ups and hyperscalers operating in unpredictable computational environments where future energy requirements are difficult to model accurately. Modularity here is not just a feature but a financial strategy for dynamic growth planning.
Is Nuclear Really the Answer to AI's Energy Problem?
Among all potential energy sources, nuclear power stands out for one critical reason: consistency. Unlike renewables, nuclear energy is not subject to weather patterns or diurnal cycles. Unlike fossil fuels, it produces no greenhouse gas emissions during operation. And unlike the fluctuating demand of residential or commercial energy use, AI data centers operate continuously, at peak load, around the clock — a perfect match for nuclear's stable base load characteristics. This makes nuclear power not just a contender but also a strong candidate for solving the energy bottleneck that AI development is fast approaching.
That said, it is important to approach this subject with realism, not idealism. While nuclear power offers the energy density and stability required to meet AI's demands, it is not without challenges — and pretending otherwise does the technology a disservice.
First and foremost, nuclear waste remains a critical concern. While the volume of waste generated is relatively small compared to other forms of energy production, its longevity and required containment are non-trivial. Long-term storage solutions must be both technically sound and politically tenable — something the industry still struggles to achieve.
Then there's the question of safety. Modern reactor designs — including microreactors such as the Aalo-1 — have made significant advances in passive safety, modularity, and deployment speed. But these benefits only hold if stringent operational standards are enforced.
Beyond Safety: Assessing the Full Environmental Footprint
Environmental impact must also be considered holistically. While nuclear energy avoids the CO₂ emissions of fossil fuels, its upstream and downstream effects — such as mining, transportation, and eventual disposal — must be factored into its overall sustainability profile. True "clean energy" status can only be claimed if we manage the entire lifecycle responsibly.
With that said, dismissing nuclear as an outdated or dangerous technology is both inaccurate and counterproductive. For the specific needs of AI infrastructure — constant, high-power loads, often in isolated locations — nuclear energy offers solutions that are unmatched by current alternatives. Renewables play a role, but they are variable, and storage remains costly. Fossil fuels are being phased out, rightly so, for environmental and geopolitical reasons. That leaves nuclear as one of the few technologies that can realistically scale with AI.
So, is nuclear the answer to AI's energy challenge?
Not in isolation — but it is one of the only answers that works at scale, in real-time, under real-world conditions. For those of us in the engineering space, that's not an ideological position. It's a practical one — and a necessary one.
For a closer look at how AI models operate — from neural networks to the hardware that powers them — see our companion article: How AI Works: From Neural Networks to Real-World Use.
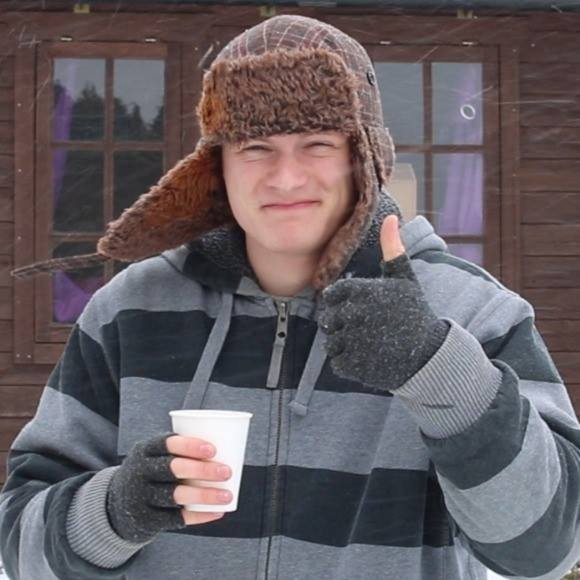