Merging Neurons with Semiconductors for Neural Interfaces
Insights | 07-05-2025 | By Robin Mitchell
The vision of directly linking the human brain to machines has long captured the imagination of scientists and technologists. In recent years, this dream has moved closer to reality, driven by breakthroughs in brain-machine interfaces (BMIs) and neurotechnology. Yet, despite the progress, researchers continue to grapple with critical challenges—chief among them, the biological and electrical incompatibilities between living neurons and synthetic circuits.
Key Takeaways:
- Breakthrough in neuromorphic hardware: Researchers have engineered a standard silicon transistor that mimics both neuron and synapse behaviours using punch-through impact ionisation.
- Single-transistor neuro-synaptic functionality: The novel configuration enables energy-efficient learning and memory within a compact CMOS footprint—critical for scalable AI applications.
- Biological compatibility insights: The transistor's activity mirrors biological plasticity, offering promising routes toward real-time, closed-loop neural interfaces.
- Implications for next-gen healthcare and computing: From neurological disorder treatment to edge AI, this technology may revolutionise how machines interface with the human brain.
What hurdles must be overcome to create seamless neuron/machine interfaces, how are researchers addressing these issues, and what does the future hold for this transformative field?
The Challenges with Neuron/Machine Interfaces
The idea of connecting the human brain to electronic circuits and computers has been around since the early 20th century, with the first experiments in the field dating back to the 1950s. However, it wasn't until the past few years that researchers have been able to make significant strides in this field, thanks to advances in technology and a deeper understanding of the human brain. One such example is the development of Neuralink, a neurotechnology company founded by Elon Musk with the goal of developing implantable brain-machine interfaces (BMIs) that would enable humans to control technology with their thoughts.
Despite the promising developments in this field, researchers face numerous challenges when it comes to creating functional neuron/machine interfaces. One of the primary challenges is that the electrical signals generated by the brain are not compatible with electronic circuits. Unlike traditional electronic components, which generate electricity through the movement of electrons, the brain uses chemical reactions to generate electrical impulses. For example, neurons use calcium ions to generate electrical signals, which are then transmitted to other neurons through specialised channels called synapses.
This means that simply connecting a wire to a neuron will not allow for direct communication between the brain and a circuit. Instead, researchers must develop specialised interfaces that can detect these chemical signals and convert them into electrical signals that can be read by a circuit. This is a complex task, as the brain generates a wide range of electrical signals, including action potentials, which are the electrical impulses that neurons use to communicate with each other.
Another challenge faced by researchers is that neurons are living cells, and as such, any connections made to them must be biologically inert. This means that any materials used to connect the brain to a circuit must be non-toxic and non-reactive, as any foreign substances could trigger an autoimmune response. This is particularly challenging when developing implantable devices, as any foreign material that is introduced into the brain can cause inflammation and damage to surrounding tissue.
The complexity of neural signals is another major challenge faced by neuron/machine interfaces. While researchers have made significant progress in understanding how individual neurons communicate, they still have a limited understanding of how large groups of neurons work together to process information. As a result, researchers are often unable to determine what specific pulses of electricity mean or how neurons arrive at decisions on a large scale.
Researchers Create New Transistors Capable of Neural Interfacing
Recently, researchers from the National University of Science and Technology (NUS) have successfully merged human neurons with semiconductive materials. This breakthrough has the potential to drastically change the way we process information, as it could lead to the development of more efficient and energy-friendly electronic devices.
To better understand the breakthrough achieved by the NUS research team, it’s helpful to visualise how conventional transistors have been adapted to replicate biological neural behaviour. The following diagram illustrates the structure and operational roles of CMOS-based transistors functioning as neurons and synapses within neuromorphic systems—a foundational step toward brain-like computing in silicon.
Illustration of CMOS transistors engineered for neuro-synaptic functionality, demonstrating their application in neuromorphic systems. Source: Nature (2025) (Click to Enlarge)
Energy-Efficient CMOS Transistors for Neuromorphic Systems
By leveraging a punch-through impact ionisation regime in standard CMOS transistors, the researchers demonstrated that synaptic and neural behaviours—including long-term potentiation, depression, and leaky-integrate-and-fire characteristics—can emerge without the need for exotic materials or complex device architectures. These findings not only eliminate the need for additional hardware layers but also present a cost-effective route for scalable neuromorphic hardware, an area crucial for edge computing and AI workloads.
The research team, led by Associate Professor Mario Lanza from the Department of Materials and Science Engineering at the College of Engineering, has developed a single silicon transistor that can function as a biological neuron and synapses when operated in a specific manner. According to the study published in the journal Nature, this transistor is highly scalable and energy-efficient, making it a promising solution for hardware-based artificial neural networks (ANN).
Unlike traditional CMOS-based approaches that require multiple transistors to emulate basic neural behaviour, this novel configuration enables neuron-like and synapse-like activity using a single transistor structure. When configured correctly, it supports multi-level synaptic weights with low variability, a feature essential for developing high-density, energy-efficient neuromorphic memory architectures.
The Role of Neuromorphic Computing in Brain-Like Electronics
Neuromorphic computing is a relatively new field that aims to develop electronic devices that mimic the brain's processing capabilities. Unlike traditional computers, which rely on complex algorithms and programming, neuromorphic devices use biologically inspired models to process information. The goal of neuromorphic computing is to create devices that can learn and adapt to new situations, much like the human brain.
Interestingly, the dynamic characteristics observed in these transistors—such as spike-timing-dependent plasticity and synaptic decay—closely mirror biological processes. This behavioural overlap reinforces their potential in future systems designed to process unstructured sensory data, where real-time adaptation and low power consumption are critical.
While the research is still in its early stages, it has the potential to drastically change the field of neuromorphic computing. If successful, this technology could lead to the development of more efficient and energy-friendly devices, which could have a notable impact on various industries, including healthcare, finance, and education. Additionally, the use of neuromorphic devices could also lead to the development of new treatments for neurological disorders, such as Alzheimer's and Parkinson's diseases.
In this context, the ability to fine-tune synaptic weights across millions of cycles with minimal drift offers tangible benefits for developing closed-loop neural interfaces. These interfaces could adapt in real time to changes in brain activity, enabling next-generation therapies for neurodegenerative conditions and improving outcomes in brain–computer interaction research.
The Future of Human/Machine Interfaces: A New Era of Connectivity
While the researchers have not yet demonstrated that their device works with a live human, their experiment with neurons presents an exciting new direction for human/machine interfaces. If the design can be successfully scaled up, it could provide a much more capable interface between humans and machines. Such a device would be able to more naturally understand the signals emanating from the brain, allowing for more intuitive and responsive interactions.
However, the potential of this technology goes beyond just improving human/machine interfaces. The development of neuromorphic devices with the ability to mimic the behaviour of biological neurons could also lead to the creation of more efficient and energy-friendly electronic devices. As the world continues to rely on electronic devices for communication, entertainment, and commerce, the demand for energy-efficient solutions is becoming increasingly important. By mimicking the brain's ability to learn and adapt, these devices could become more intelligent and responsive, leading to new applications in fields such as healthcare, finance, and transportation.
In addition to their potential applications, the development of neuromorphic computing also raises interesting questions about the future of human/machine interfaces. As machines become more intelligent and responsive to human input, the line between humans and machines will continue to blur. This raises the possibility of a future where humans are no longer the dominant force in the world but instead are integrated into a larger network of intelligent machines. While this may sound like science fiction, the rapid development of artificial intelligence and machine learning algorithms makes it a very real possibility.
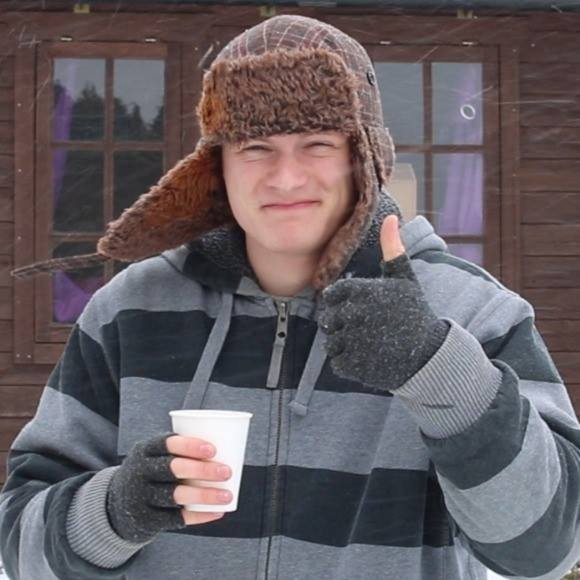