AI Helps to Identify New Types of Sclerosis in MS Patients
| 20-04-2021 | By Robin Mitchell
Recently, researchers utilised thousands of scans of MS patients and utilised AI to identify three new types of sclerosis. Why is AI ideal for medical diagnosis, how were the researchers able to utilise AI, and what challenges does AI face in the medical field?
Why is AI ideal for medical diagnosis?
AI has many uses in many fields, but it has only been the last decade that has seen exponential growth in AI technology. This recent growth has multiple contributing factors, including the development of dedicated AI hardware, widespread availability of data, and continuing research into the field, which helps develop more efficient algorithms.
The medical field is highly reliant on large data sets and identification of symptoms which are two things that AI excels in. However, the quality of medical diagnosis to patients varies dramatically across the world, and misdiagnosis and the inability to access basic medical diagnoses can lead to treatable conditions becoming either life-long complications or fatal. Furthermore, there are plenty of areas with high medical care standards but lack the staff to meet demand (such as general practitioners in the UK).
If medical diagnosis were driven by AI instead of humans, anyone would obtain a preliminary exam before being seen by doctors. Instead of waiting for an available appointment (which can be weeks away), patients would instead use an online service, enter their symptoms, and then be assigned either a GP or specialist depending on the AI diagnosis. Upon receiving a diagnosis from a specialist, the results would be fed back into the AI system which would further improve the system's performance.
Researchers Identify Three New Types of Sclerosis
To prove the effectiveness of medical AI systems, researchers recently used an AI system to help identify sclerosis in scans of MS patients. Instead of simply identifying sclerosis, the system detected three new variants that had not been recognised by medical professionals previously.
For the system to work, researchers provided the AI with over 6,000 scans of MS patients. After the results were produced, the researchers then used 3,000 other results to confirm that the system had indeed identified new types of sclerosis.
The need to identify the correct type of sclerosis in patients stems from treatment options. As MS can occur in different ways (such as cortex-led or lesion-led), each type can or may require specific treatment options. Using AI to identify the difference between sclerosis (whose difference may be too small to be noticed by humans ), could help improve lives.
What challenges does AI face in the medical field?
While almost every other industry has raced to experiment and implement AI, the medical field is one of the few that seems to be resisting its use. The exact cause for this is a multidimensional problem that includes attitudes of medical professionals, data access, and privacy.
Generally speaking, medical professionals are against the use of AI in diagnoses as doctors believe they are better able to handle patients and differentiate between important symptoms and those that are not. Furthermore, doctors also believe that they can better relate to patients and provide a human connection that a machine cannot.
AI systems require large amounts of data to work correctly, and this is something that medical AI platforms currently lack. The lack of data is not that there is little data to work with, but privacy concerns of patients and how the AI may use that data. Confidentiality of medical records is arguably one of the most important pillars of modern medicine, and such data being made available to third parties does provide valid reasons for concern. However, this may change with the introduction of new encryption methods that allow systems to affect encrypted data without needing to decrypt it first.
If bias against AI was abolished, doctors could better use computer systems to not only efficiently diagnose patients but begin to identify relationships between all kinds of lifestyle data from height, weight, alcohol intake, place of birth etc.
Read More
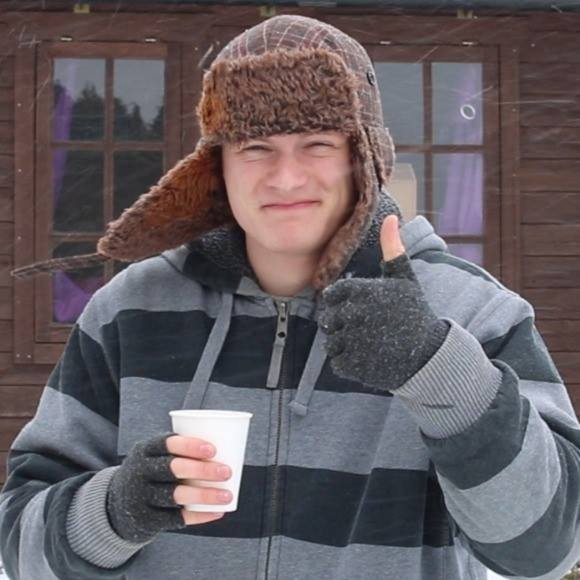