AI Tools for Fault Management
05-05-2020 | By Nnamdi Anyadike
The speed with which a malfunction can be reacted to is of great importance for production companies. In order to facilitate a more rapid response to breakdowns, manufacturers are increasingly turning to artificial intelligence (AI) as a fault management tool. In a recent paper that surveyed machine learning (ML) solutions to tackle faults in industry 4.0 the authors, from various Greek academic institutions, identified three steps in fault detection and diagnosis: data collection; data processing for feature extraction and; fault classification based on supervised, unsupervised and deep learning methods. Supervised Learning-Based Solutions (SLBS) involve the development of an automated diagnosis tool to reduce human effort and improve diagnostic accuracy. Unsupervised Learning-Based Solutions (ULBS) rely on previous knowledge and diagnostic skills by developing an intelligent fault detection scheme. This involves a two-stage unsupervised learning method using a neural network (NN); Deep Learning-Based Solutions (DLBS), meanwhile, rely on raw data and multiple layers and have recently been developed to improve the performance of fault detection and overcome the limitations of conventional ML methods.
SPAICER Project Launches to Develop Data-Driven Ecosystem
On April 28 the SPAICER project, "Scalable, adaptive production systems through AI-based resilience optimization", was launched. The aim is to use leading AI technologies and Industry 4.0 standards to anticipate disruptions and optimally adapt production planning in response. SPAICER is a consortium that includes: the German Research Center for Artificial Intelligence (DFKI), which acts as coordinator; the Machine Tool Laboratory (WZL) at RWTH Aachen University; the University of Freiburg; the Technical University of Darmstadt; the Institute for Technology and Innovation Management at RWTH; Aachen University and; the Otto Beisheim School of Management (WHU) as well as South Korean press manufacturer, SIMPAC Europe GmbH.
The partners hope to create AI-based software solution methods for industry that will enable malfunctions and changes in the integrated systems and other external factors to be predicted “in near real time.” Frank Kögler, service manager and project initiator at Simpac, explained: "With the results that we receive from the project studies, we can carry out our own tests, which we can use to take the presses to a new level technologically. The preventive detection of faults enables us to develop optimal solution modules and optimize our actions. This is an immense advantage for our customers, since possible downtimes can be almost eliminated. We are taking a big step towards the smart factory.”
SPAICER is trialling four cases. In the first, a German medium-sized company, where costs of up to Euro 500,000 per hour are incurred in tool wear and replacement, is highlighted. The project claims that by “classifying disturbances and recommending suitable measures, the stabilization and optimization of affected systems as well as the local restructuring of production processes and personnel deployment is achieved more efficiently.” In the second, SPAICER was able to optimise production planning for industrial glass production by stabilizing the supply chain through early, alternative logistics planning via roads, rail and waterways, as well as by requesting substitute suppliers of the required raw materials. In the third, SPAICER enabled a tool producer to predict the optimal time and process for cleaning or maintenance of the tools and to transfer the gained knowledge to other production lines, locations or producers. In the fourth, an automotive supplier that develops precision products for car and truck engines SPAICER was able to anticipate supply chain stoppages.
Yokogawa Releases AI-Enabled Fault Detection Device
In April, Tokyo based Yokogawa Electric Corporation announced the release of AI-enabled versions of existing devices, one of which the company says, “will help users identify and correct problems before they have a chance to cause disruptions in production operations.” The new launches are versions of the GX series panel-mount type paperless recorders; GP series portable paperless recorders; and GA10 data logging software, which are components of the SMARTDAC+TM data acquisition and control system. Recorders are used in manufacturing and R&D to acquire, display and record data on voltage, current, temperature, flow rate, pressure, and other process variables. The new AI functionality includes the ‘future pen’, a function developed by Yokogawa that enables the drawing of predicted waveforms that has been added to the GX/GP series recorders. The AI ‘future pen’ function will allow users to identify the likelihood that an alarm will occur and take action in advance. A machine learning function has been added to the GA10 data logging software to enable the detection of anomalies and the prediction of potential failures. “Data on which device is expected to fail is displayed on screen, highlighted within a yellow frame. Maintenance can thus be performed before the device fails, minimizing the likelihood of any disruptions to production,” says the company.
(top) GA10 display (bottom left to right) e-RT3 Plus and GX/GP
Credit: Yokogawa
Siemens and SAS Partner to Deliver AI-Embedded IIoT Analytics
Siemens AG, the German multinational conglomerate company, and SAS, the provider of data management and advanced analytics, recently partnered to deliver AI-embedded IIoT analytics for the edge and the cloud. The solution enhances remote monitoring, diagnostics, failure modelling, and predictive and prescriptive maintenance using Siemens’ ‘MindSphere’, the cloud-based, open IoT platform. “SAS analytics on Siemens ‘MindSphere’ solutions enable customers to improve their business with self-made edge-to-cloud IoT Analytics with embedded AI. These solutions include edge-to-cloud streaming analytics, which allows customers without specific analytics expertise to easily import, develop, train, deploy and manage analytics models onto different edge devices and into the cloud. This use case demonstrates the power of streaming analytics in remote asset performance management,” says the Siemens Web site.
Outlook
A report from Research and Markets, released in April, predicts the global smart manufacturing market will grow at a CAGR of 12.4% between 2020 and 2025 to achieve a valuation of $384.8 billion, compared with $214.7 billion in the full year 2020. Among the key growth drivers are the growing adoption of Industry 4.0; rising emphasis on industrial automation in manufacturing processes; increasing complexities in supply chains and surging demand for software systems that reduce time and cost.
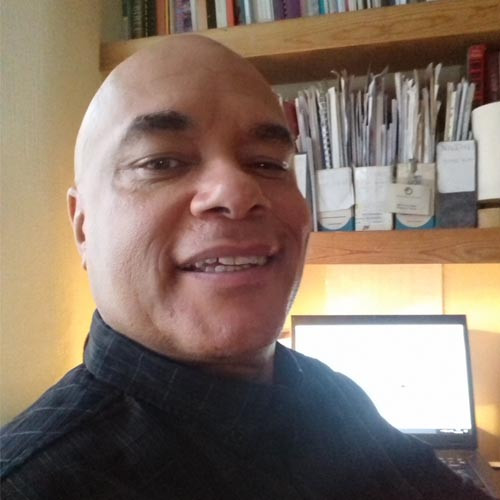