NHS AI Breakthrough: Tiny Cancer Detection Surpasses Doctor
03-04-2024 | By Robin Mitchell
Key Things to Know:
- AI diagnostic systems, like Mia, are advancing the early detection of breast cancer, demonstrating the potential to identify subtle signs of the disease that may be missed by human clinicians.
- The development and success of AI in medical diagnostics are contingent upon overcoming significant challenges, including the need for extensive, diverse, and anonymized training data, while adhering to patient confidentiality and privacy concerns.
- Regulatory, ethical, and transparency issues in AI healthcare require careful navigation to ensure the technology's responsible and beneficial integration into medical practices.
- AI's capability to provide rapid analysis could significantly impact patient care by reducing diagnostic waiting times and enabling earlier interventions, ultimately improving patient outcomes and survival rates.
As advancements in AI continue, so do its potential applications, and its ability to absorb massive amounts of data makes it prime for use in the medical field. While there are many doctors who are indifferent about AI used in diagnosis, a recent trial of an AI diagnostic system has demonstrated that AI is better at spotting early breast cancer. What challenges do AI medical diagnostics face, what did the researchers demonstrate, and how could this be game-changing for the future of breast cancer detection?
Challenges Faced by AI Medical Diagnostics
Over the past decade, artificial intelligence has seen extraordinary amounts of development, being able to identify objects in images, park cars with no one at the wheel, and even make rapid trading decisions in stock markets. Of course, such development has only been possible thanks to the immense amount of data available to researchers, as well as the increasing power of key hardware such as GPUs.
However, AI has also made significant strides in the field of medical diagnostics, particularly in the early detection of diseases such as breast cancer. However, despite the promising results, AI medical diagnostics face several challenges that need to be addressed for widespread adoption and success.
Overcoming Data Diversity and Privacy Hurdles in AI Diagnostics
One of the primary challenges faced by AI medical diagnostics is the need for extensive training data. AI algorithms require large datasets to be trained effectively, especially when identifying subtle signs of diseases like breast cancer. Obtaining diverse and anonymized data for training purposes can be a complex process due to patient confidentiality and privacy concerns. Without access to a wide range of data, AI tools may not be as accurate or reliable in detecting diseases.
Addressing the challenge of extensive training data, initiatives like NHS's AI Lab are making significant strides. The AI Lab focuses on accelerating the safe adoption of AI in health and care by facilitating access to large quantities of complex information. By bringing together government, health and care providers, and academics, the AI Lab ensures AI technologies like Mia are developed with a rich dataset, enhancing their reliability and effectiveness in clinical settings.
The regulatory landscape and ethical considerations surrounding AI in healthcare also present significant challenges. Current health regulations may limit the capabilities of AI tools, such as disabling machine learning elements that allow the tool to learn and evolve over time (due to concerns with privacy). Additionally, ensuring patient data privacy and compliance with regulatory standards is paramount when developing and deploying AI medical diagnostics, and this can add both complexity and cost to such a project.
Furthermore, the interpretability and transparency of AI algorithms pose challenges in the medical field. Healthcare professionals need to understand how AI arrives at its diagnostic decisions to trust and validate its results. Ensuring that AI algorithms are explainable and transparent in their decision-making processes is crucial for gaining acceptance and adoption among medical professionals.
The importance of explainability and transparency in AI systems is echoed by the NHS AI Lab's approach to AI development and deployment. Through their comprehensive programmes, the AI Lab ensures that AI technologies are developed with a patient-centred focus, integrating ethics from the ground up. This commitment to transparency and ethical considerations lays a solid foundation for AI tools to be trusted and widely accepted by healthcare professionals.
AI Diagnostic System Successfully Identifies Tiny Cancers Missed by Doctors
The recent trial of an AI diagnostic system, Mia, has demonstrated remarkable success in identifying tiny signs of breast cancer that human doctors missed. Mia was piloted alongside NHS clinicians and analysed mammograms of over 10,000 women, successfully flagging all cases with symptoms, including an additional 11 cases that the doctors did not identify.
Reflecting on the successful trial of Mia, it's worth noting the collaborative effort behind AI technologies in healthcare. The NHS AI Lab, through its AI in Health and Care Award, supports projects like Mia by providing essential funding and resources. This collaborative environment not only fosters innovation but also ensures that AI solutions are rigorously evaluated across multiple clinical settings, ensuring their safety, efficacy, and benefit to both healthcare professionals and patients.
Early-stage cancers can be extremely small and challenging to detect, making them easy to miss during routine screenings. The AI tool, Mia, showcased its ability to identify these minute signs of cancer, which could have significant implications for early intervention and treatment.
One of the patients, Barbara, had her 6mm tumour detected early by Mia, leading to a less invasive treatment plan that included only five days of radiotherapy. The importance of early detection was evident in Barbara's case, as breast cancer patients with tumours smaller than 15mm at diagnosis have a 90% survival rate over the following five years.
Enhancing Patient Care Through Swift AI-Powered Diagnostics
The use of AI tools like Mia not only aids in early cancer detection but also has the potential to significantly reduce waiting times for results. Mia's instant analysis could potentially cut down result waiting times from 14 days to just three, offering a faster and more efficient diagnostic process.
The potential to reduce waiting times for diagnostic results is a key advantage of AI in healthcare, as highlighted by patient advisors and experts involved in AI projects supported by the NHS. The emotional and psychological impact of waiting for a diagnosis cannot be understated. Technologies like Mia, supported by initiatives such as the NHS AI Lab, are crucial in not only improving diagnostic accuracy but also in significantly enhancing patient experience by reducing the anxiety associated with long waiting periods.
While Mia proved to be a valuable tool in identifying cancers, it is important to note that human review was still an essential part of the process. Each case analysed by Mia was also reviewed by clinicians, ensuring a comprehensive and accurate diagnosis.
Dr Gerald Lip, the clinical director of breast screening in the northeast of Scotland, highlighted the potential of AI tools like Mia to enhance the efficiency of clinical workflows. By integrating AI tools into the diagnostic process, radiologists can benefit from insights and support in identifying subtle signs of diseases.
The Future of AI in Breast Cancer Detection
The successful trial of the AI diagnostic system Mia in identifying tiny signs of breast cancer missed by human doctors marks a significant milestone in the field of medical diagnostics. Looking ahead, the implications of this technology could be game-changing for the future of breast cancer detection and patient outcomes.
The ability of AI tools like Mia to detect early signs of breast cancer that may be missed by human clinicians is crucial for improving patient outcomes. Early detection of cancer, especially when tumours are small, can lead to less invasive treatment options and higher survival rates. For example, patients with tumours smaller than 15mm at diagnosis have a 90% survival rate over the following five years. By catching cancers at their earliest stages, AI tools can facilitate prompt intervention and personalised treatment plans, ultimately improving the quality of care for patients.
One of the key advantages of AI tools like Mia is their ability to provide instant analysis of mammograms, significantly reducing the waiting time for results. Tools like Mia could potentially cut down result waiting times from 14 days to just three, offering a faster and more efficient diagnostic process. Moreover, integrating AI tools into clinical workflows can help streamline the workload of healthcare professionals. For instance, the hope is that AI tools could eventually replace one of the radiologists reviewing each scan, effectively halving the workload for each pair. This increased efficiency can lead to quicker diagnoses, reduced patient anxiety, and improved overall healthcare services.
The ability of AI tools like Mia to identify early signs of breast cancer that may be overlooked by human clinicians will undoubtedly become crucial for improving patient outcomes in the future. Early detection of cancer, especially when tumours are small, can lead to less invasive treatment options and higher survival rates, which is beneficial not only for patients but also for the NHS as a whole due to the reduced cost of care. By enabling prompt intervention and personalised treatment plans, AI tools can enhance the quality of care for patients and potentially save lives.
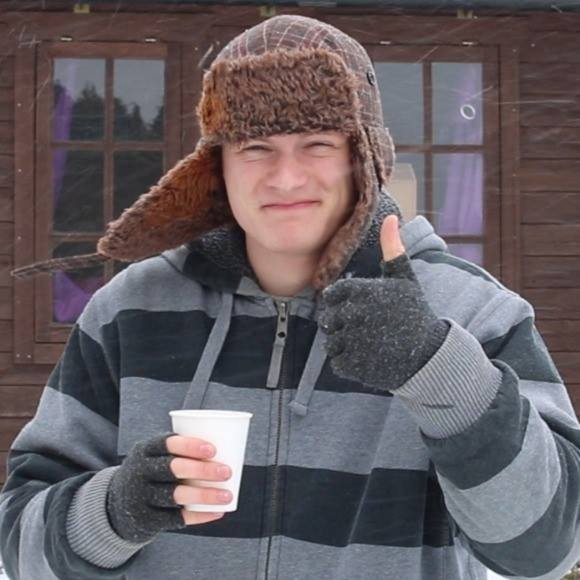