Math Formula Helps 5G
| 22-06-2020 | By Robin Mitchell
A team of researchers have developed a formula which may help to improve the performance of 5G. What does this formula do, and how is machine learning involved in the process?
Machine Learning
Machine learning is a field of engineering that has dramatically matured over the past decade thanks to the increasing power of computational systems, and availability of data. Unlike traditional systems, machine learning provides engineers with a tool that can not only be taught to recognise patterns, but also learn from their environment, which helps to improve their performance over time. During the early developments of machine learning, it was mostly used in image and speech recognition, but in recent years this is changing. Machine learning is now being used in a wide range of applications, including medical diagnostics, stock market decisions, and even environmental controls.
Channel Finding
Wireless technologies are incredibly complex, and each iteration of technology adds a whole extra layer of complexity. The first wireless technologies, based on radio blips, would use spark gaps to receive signals while the next generation of radio would use a diode to demodulate signals to extract audio information. After a few iterations of wireless technology, complex digital circuitry incorporating cryptographic functions would be deployed to keep information private. Now that many devices are moving towards mobile technologies, there is a massive demand on cell towers with potentially thousands of simultaneous connection requests. To help manage this load, radio systems deploy channels with each channel handling x number of devices and devices in one channel cannot interfere with a device in another channel. But finding a channel that has low traffic can take a while and the best channel to use can often be a factor both devices nearby and the environment. Since trial-and-error is used to select channels, there are great inefficiencies which result in increased energy consumption, and time in execution.
Introducing Machine Learning
To solve this problem a team of researchers from the National Institute of Standards and Technology (NIST), have developed a mathematical formula that behaves similarly to machine learning algorithms. In essence, the formula selects a wireless network frequency channel based on prior experience as opposed to using a trial-and-error method. Since the system will have had a chosen configuration in the past that would relate to external factors, it can be said that the same setup provides a better chance of operating. The need for such a system stems from the fact that mobile networks are deploying a solution called License Assisted Access which uses both licensed and unlicensed bands. This means that environments which utilise both Wi-Fi and Cellular devices end up competing on channels with the result a slow down in channel finding. Therefore, if both antenna (Wi-Fi and mobile), use a machine learning-like formula to find the best channel, they can operate independently to find the best solution. According to computer simulations, the formula, which maps environmental conditions such as the number of transmitters and channels present, is able to reduce the number of tries from 45,000 channels to 10 channels making it 5,000 times faster.
Conclusion
The ability for machine learning to adapt to its environment allows it to improve performance over time. Such algorithms do not need to be limited to just audio and visual applications; they can, in theory, improve any process. Thus, engineers should look to their designs and try to identify situations which involve trial and error, then see if that could be replaced with a learning algorithm.
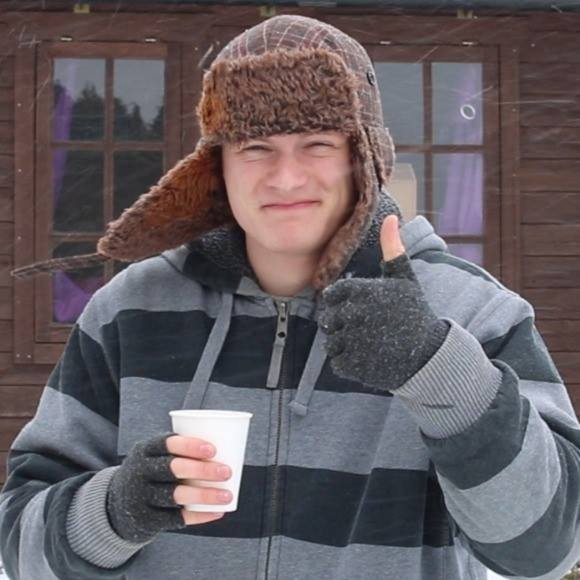