Linear Quadratic Estimation: Navigating a Way Through the Urban Canyons
23-07-2015 | By Nick Pummell
How can an 85-year-old Hungarian help car navigate the crowded streets of London, Shanghai or New York? It turns out that a talented gentleman's work in mathematics is becoming increasingly important in many different areas of transportation and navigation. The man in question is Rudolph Kalman, and fifty years ago, his work helped the US Apollo programme find its way around the world and to the Moon. Now, the Kalman algorithm for linear quadratic estimation (LQE) is a key tool for modern navigation systems, but the implementation has been giving engineers a headache ever since.
The algorithm is designed to work with unreliable data, and it uses predictions from a model of a system and maps these against the real data being used. In each cycle, this can help inherently variable systems become more accurate and is popular in navigation systems. It can also help detect changes within a system and so flag up when components fail, which is a key element of preventative maintenance.
The challenge of accurate navigation is still with us
Despite billion-dollar satellite systems providing regular positioning data, help from local cell phone networks, and mapping technology from Google, Apple and Nokia, finding exactly where you are can still be challenging. This is even more of a challenge in large cities with lots of skyscrapers that block out the satellites. This problem of the 'urban canyon' is still troubling the designers of electronic equipment for cars and trucks.
One way around this has been to rely more on mapping technology. Starting from a known point with satellite data, the turns on the map will, hopefully, show you where you are. Unfortunately, that doesn't work brilliantly well. The maps can lack accuracy, which you may know if you have followed your satnav without thinking too much about where you are going, and all the data can be time-consuming for a developer to integrate into a design. Getting more accurate maps is one of the reasons the three largest German car makers – Audi, BMW and Daimler – are set to pay $2.7bn for Nokia's map business, called Here.
What would really help is more accurate dead reckoning
This measures the turns of the vehicle via an accelerometer, combines it with the vehicle's acceleration and speed and uses the information alongside the satellite data to provide a position. Unfortunately, all these inertial sensors have errors, which may vary and can build up over time.
Enter the Kalman algorithm. This can take the sensors' errors and chart a path through the fusion of this data to provide the most accurate result.
The trouble is, at the moment, this has to be done separately for the satellite navigation system – whether it is the US GPS, Russia's GLONASS, China's BeiDou or the coming European Galileo system – and for the inertial sensors. This two-level approach creates more uncertainty, doesn't solve the problem and is very complex to implement.
Instead, what is needed is the ability to combine all this data in a single Kalman filter. This mitigates the errors from all the sources and provides the most accurate positioning data. That has been done by the designers at u-blox. The latest NEO-M8L satellite navigation module is the first to include the inertial sensors for Automotive Dead Reckoning (ADR), allowing the self-calibrating UBX-M8030 controller to implement a single Kalman filter.
This means that highly accurate satellite and dead reckoning navigation can be easily added to original and after-market automotive systems and really start to tackle the challenge of the urban canyon. All thanks to some very clever mathematics over 50 years ago.
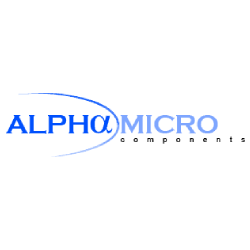